Neural computation and coding in microcircuits
One of the intellectual frontiers of modern neuroscience is to understand how thousands of neurons connected in local microcircuits combine to carry out novel and powerful computations. The Berry Lab has studied this question in the retina for many years, finding that the retina carries out a diverse set of surprisingly sophisticated computations. Of particular interest has been the ability of the retina to effectively make simple predictions about what will happen next in its visual input. Inspired by these ideas, we have recently begun to study the ability of the neocortex to carry out predictive computations. Using optical imaging in the primary visual cortex of the mouse in collaboration with the Tank lab, we have found that neurons in layer 2/3 carry out a much broader class of predictive computations in response to repeated temporal sequences. These results have then led to novel hypotheses about the different computations carried out within each layer of the neocortex which we are continuing to explore.
Complementary to these investigations is the question of how the electrical activity of neurons encodes information. While single neuron codes have long been studied, nearby neurons in microcircuits almost always have overlapping tuning curves and share correlation in their activity. This means that the fundamental coding unit is not the single neuron, but populations of hundreds of neurons or more. Using multi-electrode arrays, we have been able to record from nearly complete populations of retinal ganglion cells. And using powerful theoretical approaches, such as Maximum Entropy and Hidden Markov Models, we have been able to understand how correlation among neurons changes the structure of the neural code. We find that neural activity robustly clusters into “collective modes” of activity that represent a discrete set of population codewords. These codewords exhibit error correction and have qualitatively different receptive field properties than their constituent neurons. Our recent work suggests that this organization may be a general principle of population codes and also that this structure can be readily learned by downstream neural circuits. We plan next to test these ideas in the neocortex.
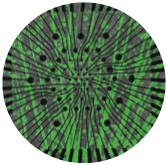